In an environment that is changing due to climate change, animal and human mobility, population growth and urbanization, the risk of new and exotic pathogens going unnoticed is increasing. Traditional infectious disease surveillance methods based on case reporting are often too slow and inaccurate. This is where the EU-H2020 project MOOD comes in. As part of the project, methods and an innovative platform were developed to improve the early detection, assessment and monitoring of infectious diseases in Europe. By using big data from a variety of sources such as geo, satellite, weather and social media data, MOOD enables a more precise and faster response to disease outbreaks.
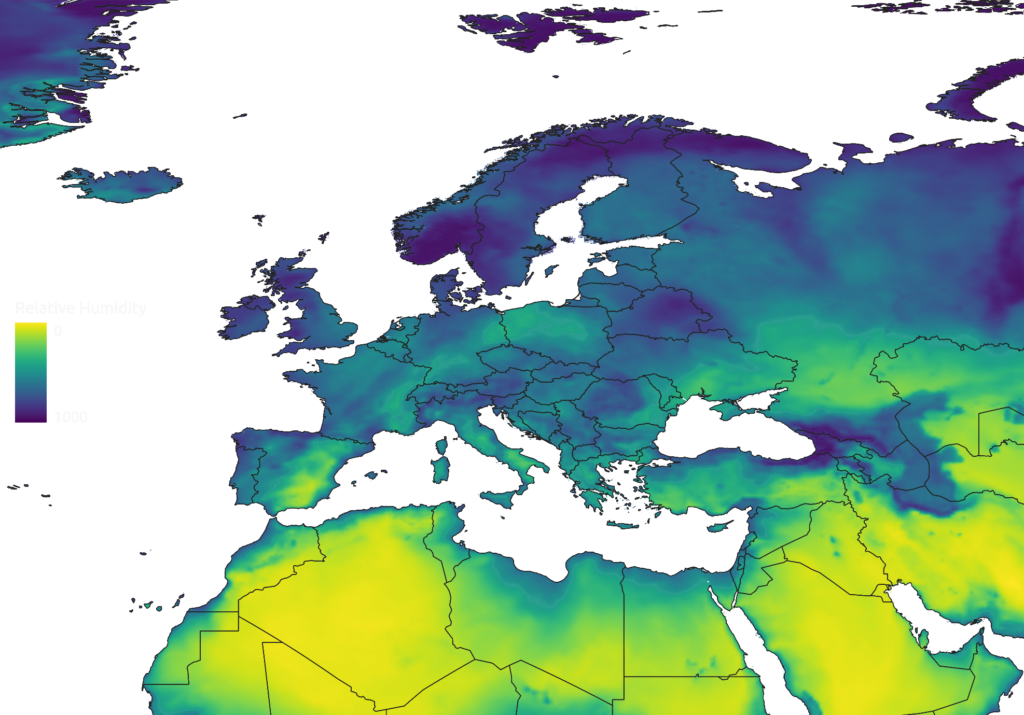